ml的問題,我們搜遍了碩博士論文和台灣出版的書籍,推薦Miner, Gary D.,Miner, Linda A.,Burk, Scott寫的 Practical Data Analytics for Innovation in Medicine: Building Real Predictive and Prescriptive Models in Healthcare and Medical 和的 Machine Learning: Theory to Applications都 可以從中找到所需的評價。
另外網站Barometers, ML-102-B, ML-102-D, ML-102-E, ML-102-F, and ...也說明:Barometers ML - 102 - D and ML - 316 / TM . ( a ) Pressure sensitive cell . ( 6 ) Lever systei ( c ) Pointer . ( 2 ) Case . ( a ) Wooden base and lid .
這兩本書分別來自 和所出版 。
國立臺北科技大學 電資學院外國學生專班(iEECS) 白敦文所指導 VAIBHAV KUMAR SUNKARIA的 An Integrated Approach For Uncovering Novel DNA Methylation Biomarkers For Non-small Cell Lung Carcinoma (2022),提出ml關鍵因素是什麼,來自於Lung Cancer、LUAD、LUSC、NSCLC、DNA methylation、Comorbidity Disease、Biomarkers、SCT、FOXD3、TRIM58、TAC1。
而第二篇論文國立陽明交通大學 電信工程研究所 吳文榕所指導 葉冠華的 汽車多雷達系統之擴展目標追蹤 (2021),提出因為有 擴展目標追蹤、形狀偵測、多雷達系統的重點而找出了 ml的解答。
最後網站Create ML 簡介:如何在Xcode 10 構建不同的機器學習模型則補充:去年,Apple 推出了Core ML,這工具讓你以最少的程式碼迅速將預先訓練好的機器 ... 如果想了解如何將Core ML 模型導入iOS App,你可以參考這篇教學。
Practical Data Analytics for Innovation in Medicine: Building Real Predictive and Prescriptive Models in Healthcare and Medical

為了解決ml 的問題,作者Miner, Gary D.,Miner, Linda A.,Burk, Scott 這樣論述:
Practical Data Analytics for Innovation in Medicine: Building Real Predictive and Prescriptive Models in Personalized Healthcare and Medical Research Using AI, ML, and Related Technologies, Second Edition discusses the needs of healthcare and medicine in the 21st century, explaining how data anal
ytics play an important and revolutionary role. With healthcare effectiveness and economics facing growing challenges, there is a rapidly emerging movement to fortify medical treatment and administration by tapping the predictive power of big data, such as predictive analytics, which can bolster pat
ient care, reduce costs, and deliver greater efficiencies across a wide range of operational functions.Sections bring a historical perspective, highlight the importance of using predictive analytics to help solve health crisis such as the COVID-19 pandemic, provide access to practical step-by-step t
utorials and case studies online, and use exercises based on real-world examples of successful predictive and prescriptive tools and systems. The final part of the book focuses on specific technical operations related to quality, cost-effective medical and nursing care delivery and administration br
ought by practical predictive analytics.
ml進入發燒排行的影片
當西瓜遇上冰淇淋!3道創意西瓜冰品
謝謝觀看,別忘了訂閲我們的頻道並分享給大家,收看我們最新發布的食譜。訂閱頻道⬇️
https://bit.ly/2vCwfMy
******************************************************
1. 西瓜冰淇淋蛋糕
份量:8人份
準備時間:20分鐘
冷凍時間:2小時
難易度:簡單
所需材料:
400 g 綠色冰淇淋(例如蘋果、薄荷或萊姆口味)
300 g 白色冰淇淋(例如優格、香草或檸檬口味)
400 g 紅色冰淇淋(例如草莓、覆盆子或櫻桃口味)
50 g 巧克力珠
此外:
26 cm 圓形扣環蛋糕模
作法:
1.1 將蘋果冰淇淋在圓形扣環蛋糕模內緣圍成一圈,冷凍約 30 分鐘。
1.2 然後將檸檬冰淇淋鋪在蘋果冰淇淋的內環中,冷凍 30 分鐘。
1.3 將草莓冰淇淋與巧克力珠混合拌勻,然後填滿圓形扣環蛋糕模中間。最後放入冰箱冷凍一小時。切西瓜冰淇淋蛋糕時,最好用加熱過的刀子,會比較好切開。
2. 西瓜裡的西瓜冰淇淋
份數:8人份
準備時間:25分鐘
冷凍時間:8小時
難易度:中等
所需材料:
1 顆紅肉小西瓜
200 g 覆盆子
350 ml 打發鮮奶油
50 g 巧克力珠
作法:
2.1 切除西瓜上蓋,用手持調理棒將西瓜裡的所有的果肉打成汁。
2.2 取出大約 1/3 的西瓜汁並加入覆盆子,再攪碎。
小秘訣:為了讓冰淇淋更滑順,最好將果汁過篩。
2.3 拌入打發鮮奶油後,將西瓜放入冰箱冷凍2小時。
2.4 用打蛋器拌入巧克力珠後,再將西瓜冷凍6 小時(最好一夜)。
3. 西瓜冰淇淋馬卡龍
份數:10份
準備時間:30分鐘
烘烤時間:15分鐘
冷卻時間:1小時30分鐘
難易度:困難
所需材料:
馬卡龍部份:
2 份 45 g 杏仁粉
2 份 75 g 糖粉
2 份 36 g 蛋白(室溫)
2 份 10 g 細砂糖
紅色和綠色食用色素
0.1 g 黑芝麻
300 g 白色冰淇淋(例如優格、香草或檸檬口味)
此外:
2 個擠花袋及花嘴
作法:
3.1 用電動打蛋器將蛋白加糖打發。小心地加入紅色食用色素,將蛋白打至堅挺。將過篩的糖粉和杏仁粉,輕輕拌入打發蛋白中。然後將打發蛋白裝入裝有花嘴的擠花袋裡。
3.2 重複上述步驟,用綠色食用色素做出綠色打發蛋白。
3.3 在烤盤上鋪上一張烘焙紙,然後將紅色打蛋蛋白用螺旋方上擠在烘焙紙上。然後在上面撒上一些黑芝麻。靜置乾燥約10至12分鐘後,將烤盤放入上下火預熱至150°C烤箱中,烤12至15分鐘。然後將烤盤取出烤箱,讓其完全冷卻。
3.4 同樣將綠色打發蛋白以螺旋狀擠在鋪著烘焙紙的烤盤上。等待乾燥10至12分鐘,然後將烤盤放入上下火預熱至150°C的烤箱中,烤12至15分鐘。然後將烤盤取出烤箱,讓其完全冷卻。
3.5 用保鮮膜將白色冰淇淋包成和馬卡龍直徑相同的圓柱形,然後放入冰箱冷凍一小時。然後將冰淇淋切成薄片。
3.6 現在來將它們組合在一起。以綠色馬卡龍為底,中間放一片白色冰淇淋,上面放一片黑芝麻朝上的紅色馬卡龍。
說到西瓜冰淇淋,當然不能錯過這道牛奶雪花西瓜冰:https://youtu.be/4FYpl6jE3ck
******************************************************
想知道更多的美味食譜嗎? 請訂閱我們的頻道,不要錯過任何新影片。有口福每天為你帶來不同的食譜影片。 與您的朋友和家人分享!
這裡可以訂閱我們的頻道:youtube.com/有口福
Facebook追蹤我們:https://www.facebook.com/yokofu.tw
Instagram追蹤我們:https://www.instagram.com/yokofu_taiwan/
An Integrated Approach For Uncovering Novel DNA Methylation Biomarkers For Non-small Cell Lung Carcinoma
為了解決ml 的問題,作者VAIBHAV KUMAR SUNKARIA 這樣論述:
Introduction - Lung cancer is one of primal and ubiquitous cause of cancer related fatalities in the world. Leading cause of these fatalities is non-small cell lung cancer (NSCLC) with a proportion of 85%. The major subtypes of NSCLC are Lung Adenocarcinoma (LUAD) and Lung Small Cell Carcinoma (LUS
C). Early-stage surgical detection and removal of tumor offers a favorable prognosis and better survival rates. However, a major portion of 75% subjects have stage III/IV at the time of diagnosis and despite advanced major developments in oncology survival rates remain poor. Carcinogens produce wide
spread DNA methylation changes within cells. These changes are characterized by globally hyper or hypo methylated regions around CpG islands, many of these changes occur early in tumorigenesis and are highly prevalent across a tumor type.Structure - This research work took advantage of publicly avai
lable methylation profiling resources and relevant comorbidities for lung cancer patients extracted from meta-analysis of scientific review and journal available at PubMed and CNKI search which were combined systematically to explore effective DNA methylation markers for NSCLC. We also tried to iden
tify common CpG loci between Caucasian, Black and Asian racial groups for identifying ubiquitous candidate genes thoroughly. Statistical analysis and GO ontology were also conducted to explore associated novel biomarkers. These novel findings could facilitate design of accurate diagnostic panel for
practical clinical relevance.Methodology - DNA methylation profiles were extracted from TCGA for 418 LUAD and 370 LUSC tissue samples from patients compared with 32 and 42 non-malignant ones respectively. Standard pipeline was conducted to discover significant differentially methylated sites as prim
ary biomarkers. Secondary biomarkers were extracted by incorporating genes associated with comorbidities from meta-analysis of research articles. Concordant candidates were utilized for NSCLC relevant biomarker candidates. Gene ontology annotations were used to calculate gene-pair distance matrix fo
r all candidate biomarkers. Clustering algorithms were utilized to categorize candidate genes into different functional groups using the gene distance matrix. There were 35 CpG loci identified by comparing TCGA training cohort with GEO testing cohort from these functional groups, and 4 gene-based pa
nel was devised after finding highly discriminatory diagnostic panel through combinatorial validation of each functional cluster.Results – To evaluate the gene panel for NSCLC, the methylation levels of SCT(Secritin), FOXD3(Forkhead Box D3), TRIM58(Tripartite Motif Containing 58) and TAC1(Tachikinin
1) were tested. Individually each gene showed significant methylation difference between LUAD and LUSC training cohort. Combined 4-gene panel AUC, sensitivity/specificity were evaluated with 0.9596, 90.43%/100% in LUAD; 0.949, 86.95%/98.21% in LUSC TCGA training cohort; 0.94, 85.92%/97.37 in GEO 66
836; 0.91,89.17%/100% in GEO 83842 smokers; 0.948, 91.67%/100% in GEO83842 non-smokers independent testing cohort. Our study validates SCT, FOXD3, TRIM58 and TAC1 based gene panel has great potential in early recognition of NSCLC undetermined lung nodules. The findings can yield universally accurate
and robust markers facilitating early diagnosis and rapid severity examination.
Machine Learning: Theory to Applications
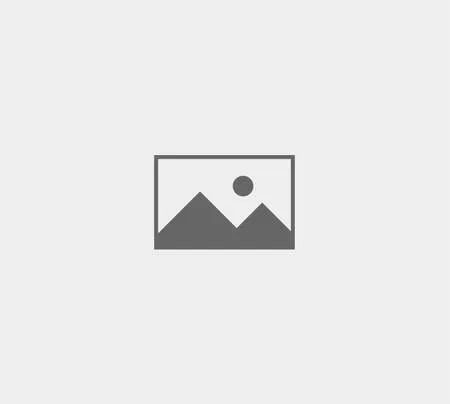
為了解決ml 的問題,作者 這樣論述:
The book reviews core concepts of machine learning (ML) while focusing on modern applications. It is aimed at those who want to advance their understanding of ML by providing technical and practical insights. It does not use complicated mathematics to explain how to benefit from ML algorithms. Un
like the existing literature, this work provides the core concepts with emphasis on fresh ideas and real application scenarios. It starts with the basic concepts of ML and extends the concepts to the different deep learning algorithms. The book provides an introduction and main elements of evaluatio
n tools with Python and walks you through the recent applications of ML in self-driving cars, cognitive decision making, communication networks, security, and signal processing. The concept of generative networks is also presented and focuses on GANs as a tool to improve the performance of existing
algorithms.In summary, this book provides a comprehensive technological path from fundamental theories to the categorization of existing algorithms, covers state-of-the-art, practical evaluation tools and methods to empower you to use synthetic data to improve the performance of applications.
汽車多雷達系統之擴展目標追蹤
為了解決ml 的問題,作者葉冠華 這樣論述:
本論文主要考慮在道路環境中之擴展目標追蹤問題。在擴展目標追蹤中,必須估計運動學及形狀兩種參數。在道路上的目標不會是任意形狀的,通常有數個特定形狀作為候選。為了提高追蹤效能,我們首先提出將形狀估計問題轉換為形狀偵測的問題。計算候選形狀的似然函數,並使用最大似然 (maximum likelihood, ML) 原理進行偵測。一旦偵測到形狀,接著再次使用 ML來估計目標位置,並將結果與追蹤濾波器的輸出相結合。使用上述方法,我們進一步提出了兩種多雷達擴展目標追蹤方法,分散式和集中式方法。由模擬結果所示,所提出的方法明顯優於傳統的擴展目標追蹤方法。
想知道ml更多一定要看下面主題
ml的網路口碑排行榜
-
-
#2.ML - 一般情況單位符號字母用小寫 - 華人百科
1m3 >1 dm3 =1 L >1 cm3 = 1mL ,每一級之間的進率都是1000 。 1升=1立方分米=1000立方釐米=1000毫升. 相關詞條. ML ... 於 www.itsfun.com.tw -
#3.Barometers, ML-102-B, ML-102-D, ML-102-E, ML-102-F, and ...
Barometers ML - 102 - D and ML - 316 / TM . ( a ) Pressure sensitive cell . ( 6 ) Lever systei ( c ) Pointer . ( 2 ) Case . ( a ) Wooden base and lid . 於 books.google.com.tw -
#4.Create ML 簡介:如何在Xcode 10 構建不同的機器學習模型
去年,Apple 推出了Core ML,這工具讓你以最少的程式碼迅速將預先訓練好的機器 ... 如果想了解如何將Core ML 模型導入iOS App,你可以參考這篇教學。 於 www.appcoda.com.tw -
#5.ML Study Jam 機器學習培訓計劃- 初級- 首頁 - Google
ML Study Jam 是Google Developers 所設計的免費機器學習培訓計劃。在這個初級培訓課程中,參加培訓的學員僅須運用Qwiklabs 線上學習平台,按照自己的步調及時間, ... 於 events.withgoogle.com -
#6.何謂機器學習? - Trend Micro
機器學習(ML) 是人工智慧(AI) 的一種,讓系統從資料當中反複學習,其方法是透過各種演算法來識別資料中的模式,然後使用可產生精確模型的資料來加以訓練, ... 於 www.trendmicro.com -
#7.連滴妹都錯?毫升ml不唸「莫」 留美網友:這樣唸才對
夏天氣溫飆高熱到汗如雨下,不少人會買冰涼的飲料消暑。據了解,飲料包裝上標示的容量單位「毫升(ml)」,多數台灣人都習慣唸成「莫」、「mo」的音, ... 於 today.line.me -
#8.Unity ML-Agents Toolkit - GitHub
The Unity Machine Learning Agents Toolkit (ML-Agents) is an open-source project that enables games and simulations to serve as environments for training ... 於 github.com -
#9.Point ML - Mali
Login to Mon Point ML. arrow. English. Register Domain. Register your FREE .ML Domain Name. Register a domain name. Point ML. Home · WHOIS. Company. 於 www.point.ml -
#10.DOME-ML
DOME-ML (or simply DOME) is an acronym standing for Data, Optimization, Model and Evaluation in Machine Learning. DOME is a set of community-wide guidelines ... 於 dome-ml.org -
#11.ML在線翻譯 - 海词
ML 的英文翻譯是什麼意思,詞典釋義與在線翻譯:. 詳盡釋義. abbr. (縮略詞). =millilambert 毫郎伯; =machine language ... 於 dict.cn -
#12.(ML音樂餐廳)我愛餐廳 - yes123求職網
品牌名稱: ML音樂餐廳; 行業類別: 餐廳/餐館; 企業電話: 07-2270177; 企業地址: 高雄市苓雅區和平一路248號2樓; 相關連結: 商業司登記|Google ... 於 www.yes123.com.tw -
#13.MORITEX - ML系列-肯定資訊科技有限公司
MORITEX - ML系列. ML系列. 規格表. 型號, 工作距離(mm), 倍率(x), 晶片尺寸(mm), 解析度(μm), 景深(mm), 失真(%), 相機接口, 型錄. ML-0614, 1/2". ML-0813, 2/3". 於 www.surevision.com.tw -
#14.體積單位毫升「mL」是「milliliter」的簡寫,別再唸作「莫」了
炎炎夏日走進超商買飲料,貨架上處處可見容量計量單位毫升(mL),多數台灣人喜歡讀成「莫」、「mo」或「more」的音,就連知名英語教學YouTuber「滴妹」( ... 於 www.facebook.com -
#15.MLCommons
It creates opportunities by putting ML in the hands of more people. MLCube isn't a new framework or service; MLCube is a consistent interface to machine ... 於 mlcommons.org -
#16.毫升_百度百科
毫升(Milliliter),符号mL,拼音为háo shēng,是一个容积单位。 於 baike.baidu.com -
#17.什麼是機器學習(ML)?為何機器學習很重要? - NetApp
A subset of artificial intelligence (AI), machine learning (ML) is the area of computational science that focuses on analyzing and interpreting patterns and ... 於 www.netapp.com -
#18.Mobile Legends: Bang Bang
11.08. KR. 1. : 2. SG · 11.08. TW. 0. : 3. MY · 11.08. ID. 1. : 2. PH. 於 m.mobilelegends.com -
#19.卜邁維斯®0.25 mmol/ml 注射液 - 台灣拜耳
產品中文名卜邁維斯®0.25 mmol/ml 注射液產品英文名Primovist® 許可證字號衛署藥輸字第024676 號本藥限由醫師使用適應症適用於T1加權掃描核磁造影時, ... 於 www.bayer.com.tw -
#20.Core ML | Apple Developer Documentation
Core ML applies a machine learning algorithm to a set of training data to create a model. You use a model to make predictions based on new input data. 於 developer.apple.com -
#21.ML(容積單位) - 中文百科全書
換算方法milliliter的縮寫,毫升,計量單位。進率:1L(升)=1dm(立方分米)= 0.001m(立方米)1mL(毫升)=1cm(立方厘米)= 0.001dm(立方分米)1m >1 dm =1 L >1 cm = 1mL ... 於 www.newton.com.tw -
#22.Monna Lisa ML-32000 | 紡織數位印花機| 大尺寸印表機 - EPSON
... 對稱式噴印技術,在保持高產能的同時,精美呈現印花品質的細膩度; 超過39年在地專業服務團隊,提供產業客戶所需的維修服務. ML-32000 產品型錄. 於 www.epson.com.tw -
#23.neptune.ai | ML metadata store
Manage all your model-building metadata in a single place. Track experiments, register models, integrate with any MLOps tool stack. 於 neptune.ai -
#24.機器學習(ML)定義為何?演算法有哪些? - OOSGA
機器學習(ML)定義為何?演算法有哪些? 最後更新於:August 28, 2022. 定義. 機器學習為何? 機器學習,一種人工智慧的技術,不同於傳統程序,是通過處理並學習龐大 ... 於 zh.oosga.com -
#25.ml - Wiktionary
SymbolEdit. ml. (metrology) Symbol for millilitre, an SI unit of fluid measure equal to 10 ... 於 en.wiktionary.org -
#26.SYS-510T-ML | Mini-1U | SuperServer | Products - Supermicro
X12 Cloud solution. Web Hosting. Single Socket H5 (LGA-1200); supports Intel Xeon E-2300 series, 10th Gen. Pentium processors; Memory Capacity: 4 DIMM ... 於 www.supermicro.com -
#27.透過機器學習(ML)驅動的大數據分析為晶片設計取得可行見解
Synopsys DesignDash 技術深入的增強分析也能實現指示性導引,為團隊提供不同的方法來解決自動辨識出來的問題(圖3)。以統計和ML 為基礎的模型,可以擷取這個工具解決特定 ... 於 www.synopsys.com -
#28.【機器學習平臺再進化:端到端MLOps】從ML平臺邁向ML生態 ...
為了大規模發展AI,3年前,Line就開始打造機器學習平臺MLU,逐漸涵蓋到全部ML訓練流程,後續更延伸到部署、服務和監控功能,現在甚至推出了互動介面, ... 於 www.ithome.com.tw -
#29.奇美CHIMEI 24型無邊框超廣視螢幕ML-24P20F
奇美CHIMEI 24型窄邊框HDR螢幕ML-24P20F / 24 1920 FHD 75Hz. $2,688. 折價券. 窄邊框-超 ... 於 24h.pchome.com.tw -
#30.ml中文(繁體)翻譯:劍橋詞典
ml 翻譯:毫升(millilitre 的縮寫)。了解更多。 ... ml 在英語-中文(繁體)詞典中的翻譯. ml. noun. written abbreviation for millilitre UK. 於 dictionary.cambridge.org -
#31.Full Power and Limited Endurance Test of the ML-1 Nuclear ...
Following a modification and checkout , the ML - l power plant operated successfully for 101 hours in February and March 1963 , B. THE ML - 1 The ML - 1 is ... 於 books.google.com.tw -
#32.Comet ML - Build better models faster
Track, compare, and reproduce your ML experiments with Comet's machine learning platform. Leverage insights to build better models, faster. 於 www.comet.com -
#33.Standard ML of New Jersey
Standard ML of New Jersey (abbreviated SML/NJ) is a compiler for the Standard ML '97 programming language with associated libraries, tools, ... 於 www.smlnj.org -
#34.Machine Learning | Android Developers
Create smarter apps. On-device machine learning (ML) lets you supercharge your app and add features to process images, sound, and text. Follow ... 於 developer.android.com -
#35.Machine Learning (ML) in IoT - Silicon Labs
Machine learning (ML) in IoT is increasingly leveraged because of the benefits it offers edge device developers. We can help you bring ML to the tiny edge. 於 www.silabs.com -
#36.轉換體積, 毫升 - Convertworld.com
使用這個簡單的工具快速轉換毫升作為體積的單位. 於 www.convertworld.com -
#37.The Future of Network Security: Predictive Analytics and ML ...
ML -driven network security solutions in cybersecurity refer to the use of self-learning algorithms and other predictive technologies ... 於 thehackernews.com -
#38.ML - yhkao
(0) ML-00: 人工智慧概論01, 前言和歷史(35:43) ... (3) ML-03: How to run Python Programs in Jupyter Notebook and Spyder (37:03). 於 sites.google.com -
#39.機器學習與人工智慧 - AWS
與Amazon 專家互動. Amazon Machine Learning Solutions Lab 讓您的團隊與Amazon ML 專家合作,為您的業務建置新的ML 解決方案。 於 aws.amazon.com -
#40.毫升- 維基百科,自由的百科全書 - Wikipedia
毫升是容量計量單位,符號為mL(為milliliter縮寫,音/ˈmɪl·əˌli·t̬·ər/,縮寫音/ mil/),又稱公撮,相當於立方公分(cubic centimeter,簡稱cc)。 於 zh.wikipedia.org -
#41.連滴妹都錯!99%台灣人把ml唸成「莫」神人解:這樣唸才對啦
在飲料、化妝品的瓶身上,都會標示容量單位「毫升」(mL),許多台灣人都會習慣把它唸成「莫」、「mo」或「more」,但其實這根本是錯誤的用法,連教英文的 ... 於 dailyview.tw -
#42.「Ml」找工作職缺-2023年2月 - 104人力銀行
2023/2/21-1886 個工作機會|ML Python工程師(FJ)【德義資訊股份有限公司】、AOI/AI/ML訓練員/研發人員【品元企業股份有限公司】、機器學習工程師AI/ML Engineer(Data ... 於 www.104.com.tw -
#43.「滴妹」也唸錯!毫升mL的英文其實這樣唸… - 生活 - 自由時報
飲料瓶身上所標示的毫升(mL),大多數台灣人習慣唸成「莫」,就連知名英文教學YouTuber「滴妹」都冠伶也這樣唸,但事實上「莫」的唸法是錯的! 於 news.ltn.com.tw -
#44.7 Must-Have Python Tools for ML Devs and Data Scientists
7 Must-Have Python Tools for ML Devs and Data Scientists. Python has an easy learning curve, however there are a range of development tools ... 於 thenewstack.io -
#45.Replicate wants to take the pain out of running and hosting ML ...
Replicate, a startup developing a platform to host and run machine learning models, has emerged from stealth with over $17 million in ... 於 techcrunch.com -
#46.【機器學習】入門介紹-什麼是機器學習What's ML?
一文中,已經簡單介紹過人工智慧(Artificial Intelligence, AI)、機器學習(Machine Learning, ML)、深度學習(Deep Learning, DL)彼此之間的愛恨 ... 於 jason-chen-1992.weebly.com -
#47.Machine Learning Library (MLlib) Guide - Apache Spark
Machine Learning Library (MLlib) Guide · ML Algorithms: common learning algorithms such as classification, regression, clustering, and collaborative filtering ... 於 spark.apache.org -
#48.Runway - Everything you need to make anything you want.
Explore more than 30+ AI powered creative tools to ideate, generate and edit content like never before. 於 runwayml.com -
#49.将毫升换算为升(ml换算为l) - 换算度量单位/ 单位计算器
1毫升等于多少升? 可以将ml换算为l (毫升换算为升)的度量计算器。 (体积) 於 www.huansuan-danwei.info -
#50.MLBB BEST TIKTOK VIDEO | ML MEMES - YouTube
Download Tongits Go To Win Ml Diamonds & Loads https://tongitsgo5.page.link/Kielzee-PlayzHow to Convert Go Stars to ML Diamonds ... 於 www.youtube.com -
#51.毫升(ml)、立方公分(c.c.)如何換算? - 黃大偉理財研究室
毫升(ml)是『容量』或『液體的體積』的計量單位,英文為milliliter, 縮寫ml,又稱為公撮,相當於立方公分。 · ✓ 1公升=1000毫升; · ✓ 1毫升=0.001公升=1立方公分=1c ... 於 davidhuang1219.pixnet.net -
#52.Evolution of ML Fact Store. by Vivek Kaushal - Netflix TechBlog
At Netflix, we aim to provide recommendations that match our members' interests. To achieve this, we rely on Machine Learning (ML) ... 於 netflixtechblog.com -
#53.mL 與cc
mL 與cc有什麼差別? 好像差不多?是嗎? mL 是milli-Liter (一公升的千分之一). cc 是cubic centimeter (立方公分). 參考維基百科. 公升,通常簡稱為升,是容量計量 ... 於 1234.ped.cc -
#54.Machine Learning (2022 Spring)
Date Topic Preparation ‑ zh Preparation ‑ en 2/18 Lecture 1:Introduction of Deep Learning 影片1 · 影片2 Video 1 · Video 2 3/04 Lecture 3:Image as input 影片 Video 3/11 Lecture 4:Sequence as input 影片1 · 影片2 Video 1 · Video 2 於 speech.ee.ntu.edu.tw -
#55.Home - Applied ML Summit - Google Cloud Webinars
The Google Cloud Applied ML Summit brought together the world's leading professional machine learning (ML) engineers and data scientists to ... 於 cloudonair.withgoogle.com -
#56.Wealth Management and Financial Services from Merrill Lynch
Merrill Lynch Wealth Management has the financial advisors and expertise to help you achieve your goals at any stage of your life or career. 於 www.ml.com -
#57.Multilevel Modeling of Secure Systems in QoP-ML
The QoP-ML is intended to represent a series of steps described as a cryptography protocol. The multilevel protocol introduced in the QoP-ML makes it ... 於 books.google.com.tw -
#58.ML-34C30Q - 奇美家電
ML -34C30Q. 21:9超寬曲面電競螢幕. 於 electronics.chimei.com.tw -
#59.Machine Learning authors/titles recent submissions - arXiv
Subjects: Machine Learning (stat.ML); Artificial Intelligence (cs.AI); Machine Learning (cs.LG); Statistics Theory (math.ST). [10] arXiv:2302.09521 [pdf, ... 於 arxiv.org -
#60.Unity Machine Learning Agents
Unity Machine Learning Agents · Create an intelligent game experience · Create realistic and complex AI environments to train models · How Unity ML-Agents works. 於 unity.com -
#61.ml the Max of Life |寶易生技股份有限公司
ml 是生活的態度,也是對品質最大要求的可能,全產品採用食用級香料、色素,原料皆可於自然界生物分解的原料,全數委由台灣檢驗科技(股)公司(SGS)檢驗,且皆經過田野 ... 於 www.more-ml.com -
#62.ML mlife - 綠界
... 如果用嗅覺, 世界會非常不一樣! 氣味會帶你走, 那是眼睛看不到, 耳朵聽不到, 而心所想像不到的美好! 深呼吸… ML mlife2020 #嗅覺是一種幸福能量#無所不在. 於 mlife2020.cashier.ecpay.com.tw -
#63.Ml Definition & Meaning - Merriam-Webster
What does the abbreviation ML stand for? Meaning: milliliter. 於 www.merriam-webster.com -
#64.What is BigQuery ML? - Google Cloud
BigQuery ML empowers data analysts to use machine learning through existing SQL tools and skills. Analysts can use BigQuery ML to build and evaluate ML models ... 於 cloud.google.com -
#65.什麼是Auto ML?又有什麼樣的特點? - 數位時代
「若用一句話形容,Google的Cloud Auto ML是一種『自動建模』工具。」玉山金控科技長暨臺灣人工智慧學校執行長陳昇瑋說。 於 www.bnext.com.tw -
#66.Made With ML: Home
Learn how to responsibly develop, deploy & maintain ML. 於 madewithml.com -
#67.使用另購的ML-L3 遙控器
從距離5 m 或更近的地方,將ML-L3 上的發射器對準相機上的紅外線接收器,然後按下ML-L3 快門釋放按鍵。在延拍遙控模式下,快門釋放前自拍指示燈將會點亮約2 秒。在即拍遙控 ... 於 onlinemanual.nikonimglib.com -
#68.What Is Machine Learning and Why Is It Important? - TechTarget
Machine learning (ML) is a type of artificial intelligence (AI) that allows software applications to become more accurate at predicting outcomes without ... 於 www.techtarget.com -
#69.什麼是AI和ML?為什麼AI產品管理比一般軟體更困難? - Medium
哪些不是?),然後建立ML模型或神經網絡,讓機器自行學習,摸索出規則。 (source: IBM Research Blog). 你和你的團隊要做 ... 於 medium.com -
#70.1000 AICTE Virtual Internships in AI/ML For Engineers - Unstop
AICTE launches 1000 virtual internships for engineers in AI/ML domain. The last date to apply- 31 March 2023, check out the eligibility ... 於 unstop.com -
#71.LINEA ML CMOS多線掃描相機| 線掃相機| 產品資訊
Linea ML CMOS多線掃描相機. 高速成像,在8K/16K解析度下,線速度高達300 kHz 具有單輸出或雙輸出的單色/HDR成像使用順序曝光在單次掃描中進行時分多場成像 於 www.linx.tw -
#72.Index of /ml/machine-learning-databases
Index of /ml/machine-learning-databases · Parent Directory · 00000/ · 00192/ · 00193/ · 00194/ · 00195/ · 00196/ · 00197/ ... 於 archive.ics.uci.edu -
#73.ML Ops: Machine Learning Operations
Getting started. Being an emerging field, MLOps is rapidly gaining momentum amongst Data Scientists, ML Engineers and AI enthusiasts. Following this trend, the ... 於 ml-ops.org -
#74.ML Kit | Google Developers
ML Kit is a mobile SDK that brings Google's on-device machine learning expertise to Android and iOS apps. Use our powerful yet easy to use Vision and ... 於 developers.google.com -
#75.連滴妹都錯!毫升ml不是唸「莫」 留美神人:這樣唸才對
許多飲料瓶身上都會標示的容量單位「毫升」(ml),大多數台灣人都習慣把它唸成「莫」,就連知名英文教學Youtuber「滴妹」(都冠伶)也這樣唸,不過這 ... 於 tw.yahoo.com -
#76.Artificial intelligence (AI) vs. machine learning (ML)
In this article, we discuss artificial intelligence, machine learning and their applications. We also explore some of their key similarities ... 於 venturebeat.com -
#77.台大資訊深度學習之應用| ADL 1.1: What is ML? 甚麼是機器學習?
2022/02/14 Applied Deep LearningLectured by Yun-Nung Vivian Chen 陳縕儂@ NTU CSIESlides credited from Hung-Yi Lee. 於 www.youtube.com -
#78.Game ML, 線上商店| 蝦皮購物
APEX ML-使用世界前沿的AI 偵測技術實現0封號的超人類反應GAME ML團隊以XBOX手把輔助瞄準作為發想出發,致力於研發鍵鼠的AI輔助瞄準工具,AI輔助並不能像一般常見的 ... 於 shopee.tw -
#79.【Arm 專欄】一表了解AI 人工智慧與ML 機器學習 - INSIDE
常常聽到「人工智慧」、「機器學習」、「深度學習」,這些好像都跟AI 有關,但到底分別代表什麼呢?#趨勢,評論,人工智慧,AI,機器學習,ML ... 於 www.inside.com.tw -
#80.The ML-1 Design Report - 第 1 頁 - Google 圖書結果
THE ARMY GAS - COOLED REACTOR SYSTEMS PROGRAM THE ML - 1 DESIGN REPORT I. INTRODUCTION This design report describes the mobile nuclear power plant being ... 於 books.google.com.tw -
#81.3种方法来把毫升(MI)转为克 - wikiHow
方法1. 方法1 的3: 理解前缀和标准单位的意义 · 克(g)是国际质量单位中的质量单位,定义就是1立方厘米(cm³) 4°C的水的质量。缩写是g · 毫升(Milliliter)则是体积单位,Ml ... 於 zh.wikihow.com -
#82.Google 機器學習三大服務:AutoML, Cloud ML Engine, ML ...
一篇文章帶您了解AutoML, Cloud ML Engine, ML API Google 在1/17. 於 ikala.cloud -
#83.ProLiant ML 伺服器 - HPE Store
HPE ProLiant ML30 Gen10 Plus 伺服器是功能強大且經濟實惠的直立式伺服器,專為小型辦公室、遠端辦公室和分公司而設計,可執行內部部署與混合雲解決方案,以低成本提供 ... 於 buy.hpe.com -
#84.M Lhuillier Financial Services
There are now over 3000 branches pursuing M Lhuillier's principle of service excellence and total customer satisfaction. 於 mlhuillier.com -
#85.機器學習教育課程 - TensorFlow
Hands-on Machine Learning with Scikit-Learn, Keras, and TensorFlow. 作者:Aurélien Géron ... Intro to TensorFlow for AI, ML, and Deep Learning. 於 www.tensorflow.org -
#86.擁有LinkedIn 檔案的Amit Marathe
XGBoost was one of my favorite ML algorithms at the time and I remember using it in a few of my submissions. Kaggle joined Google back in 2017, ... 於 tw.linkedin.com -
#87.ML Kit for Firebase - Google
ML Kit is a mobile SDK that brings Google's machine learning expertise to Android and iOS apps in a powerful yet easy-to-use package. Whether you're new or ... 於 firebase.google.com -
#88.ML for the Working Programmer - 第 xiii 頁 - Google 圖書結果
As luck would have it , changes to ML have come about at the same time . ML has a new standard library and the language itself has been revised . 於 books.google.com.tw -
#89.Artificial Intelligence and Machine Learning (AI/ML) - FDA
The FDA has updated the list of AI/ML-enabled medical devices marketed in the United States as a resource to the public. 於 www.fda.gov -
#90.Machine Learning Specialization - Coursera
Offered by DeepLearning.AI and Stanford University. #BreakIntoAI with Machine Learning Specialization. Master fundamental AI concepts and . 於 www.coursera.org -
#91.What is Machine Learning? | IBM
Machine learning is a branch of artificial intelligence (AI) and computer science which focuses on the use of data and algorithms to imitate the way that ... 於 www.ibm.com -
#92.Vivado ML Standard - Xilinx
Download Vivado™ ML Standard Edition free. Purchase licensing options for Enterprise Edition start at $2995. 於 www.xilinx.com -
#93.EDS-2016-ML 系列- 非網管型交換器 - Moxa
此外,為了針對不同產業的應用提供更多的彈性,EDS-2016-ML 系列允許使用者透過外部面板DIP 開啟或停用服務品質功能(QoS),廣播風暴防護和連接埠中斷警報功能。 除了其小巧 ... 於 www.moxa.com -
#94.ClearML: MLOps for Data Scientists, ML Engineers, and DevOps
Easily Develop, Orchestrate, and Automate ML workflows at scale. ClearML's open-source MLOps platform is your end-to-end solution. Start free! 於 clear.ml -
#95.Modern Compiler Implementation in ML - 第 29 頁 - Google 圖書結果
( * ML Declarations : * ) type lexresult fun eof ( ) % 88 % = ( * Lex Definitions : * ) digits = [ 0-9 ] + = Tokens. 於 books.google.com.tw -
#96.Definition of mL - NCI Dictionary of Cancer Terms
A measure of volume in the metric system. One thousand mLs equal one liter. Also called cc, cubic centimeter, and milliliter. 於 www.cancer.gov -
#97.什麼是自動化機器學習(AutoML)? - Azure - Microsoft Learn
您可以透過Azure ML Python SDK 來支援撰寫視覺工作的AutoML 模型。 產生的實驗作業、模型和輸出可以經由Azure Machine Learning 工作室UI 進行存取。 於 learn.microsoft.com